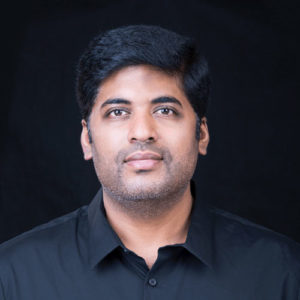
Dheeraj Nallagatla
Founder & CEO
Artificial Intelligence (AI) is not just a buzzword; it is the culmination of decades of dreams, research, and relentless pursuit of progress. To understand how AI has evolved into the transformative force we know today, we need to trace its journey from its inception to its promising future.
The Dawn of AI: When Vision Met Innovation
The concept of machines that could mimic human intelligence dates back to ancient myths and philosophical musings. There are several examples of artificial beings or forms of artificial life in ancient myths, like “The myth of Talos” from Greek mythology and “The Golem” from Jewish Folklore.
However, AI as a formal field began in 1956 when John McCarthy, Marvin Minsky, Nathaniel Rochester, and Claude Shannon organized the Dartmouth Conference. This historic meeting laid the groundwork for defining AI as the science and engineering of creating intelligent machines. John McCarthy is the computer scientist who coined the word “Artificial Intelligence” in 1956.
In the early years, pioneers like Alan Turing introduced the idea that machines could perform tasks requiring “intelligence” through his famous Turing Test. Despite these early theoretical breakthroughs, progress was slow due to the limitations of computing power and the complexity of programming. The AI winters—periods where funding and interest declined significantly due to unmet expectations and technology limitations—were testaments to these struggles.
The Rise of Machine Learning: A Shift in Approach
The 1980s and 1990s brought a pivotal shift. Researchers began focusing on data-driven approaches, moving from hard-coded logic to systems that could learn from experience. This shift marked the birth of machine learning (ML), where algorithms could identify patterns and make decisions without explicit programming. Techniques such as neural networks, inspired by the human brain, gained attention but initially faced limitations due to computational constraints.
The breakthrough came in the 2010s when an abundance of data, powerful GPUs, and refined algorithms catapulted AI into mainstream use. Deep learning, a subset of machine learning involving multi-layered neural networks, enabled machines to achieve unprecedented feats such as understanding images, processing natural language, and even playing complex games like Go at superhuman levels.
The Role of Cloud Computing in AI’s Growth
One of the major catalysts for the rapid growth of machine learning and AI in recent years has been cloud computing. The advent of cloud platforms provided scalable, cost-effective infrastructure that allowed researchers and companies to access vast computational resources without the need for expensive, on-premise hardware. Services offered by providers such as Amazon Web Services (AWS), Microsoft Azure, and Google Cloud democratized access to powerful processing capabilities and data storage.
Cloud computing supported the development of AI by enabling large-scale data processing and model training. This shift meant that even smaller organizations could run complex machine learning models, experiment with algorithms, and deploy AI solutions at scale. Additionally, the cloud’s ability to integrate machine learning frameworks and tools streamlined the workflow for data scientists, boosting productivity and fostering innovation.
AI Today: The Era of Smart Systems
Today, AI is embedded in our daily lives in ways that were once the realm of science fiction. From voice assistants like Siri and Alexa to personalized recommendations on streaming platforms, AI is everywhere. In businesses, AI optimizes supply chains, enhances customer interactions, and drives predictive analytics that unlock deeper insights.
Technologies like Large Language Models (LLMs), such as ChatGPT or Gemini, showcase how far natural language processing has come. These systems are capable of holding human-like conversations, generating code, and assisting in creative tasks. Healthcare, finance, and education are just a few sectors witnessing dramatic transformations due to AI’s capabilities.
Understanding AI, Machine Learning, Deep Learning, Foundational Models, and LLMs
To grasp the current state and future of AI, it’s important to differentiate between key concepts:
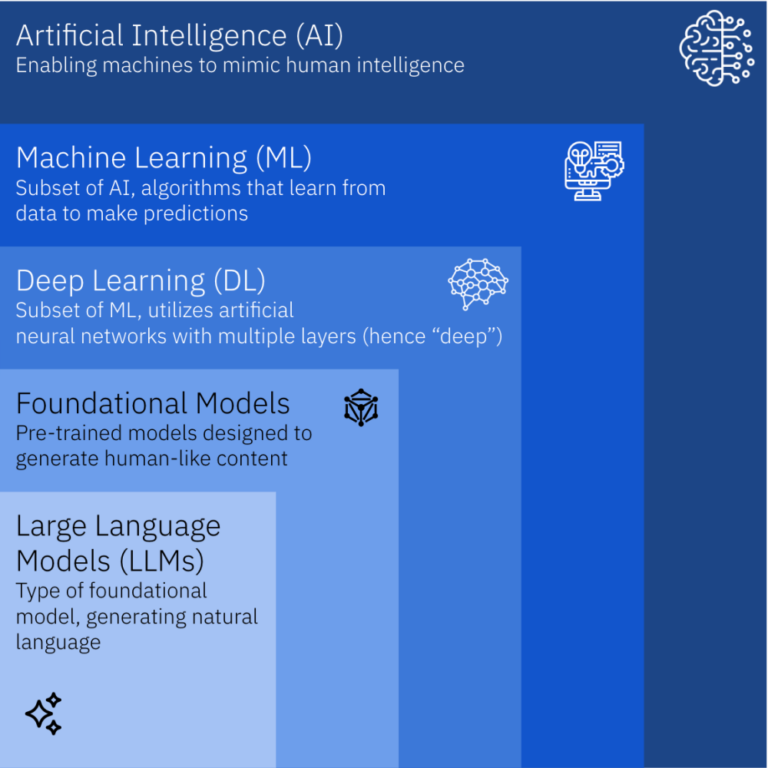
- Artificial Intelligence (AI): The overarching field that encompasses any technique enabling machines to mimic human intelligence. It includes everything from simple rule-based systems to complex machine learning algorithms.
- Machine Learning (ML): A subset of AI focused on algorithms that learn from data to make predictions or decisions. Unlike traditional programming, ML systems improve their performance as they are exposed to more data over time.
- Deep Learning: A specialized subset of machine learning that utilizes artificial neural networks with multiple layers (hence “deep”). Deep learning excels at handling large datasets and complex tasks such as image recognition, natural language processing, and speech synthesis.
- Foundational Models: These are large, pre-trained models designed to understand and generate human-like text, images, or other types of data. Foundational models are trained on massive datasets and can be fine-tuned for specific applications, forming the basis for many advanced AI tools.
- Large Language Models (LLMs): A type of foundational model specifically trained on extensive text data to understand and generate natural language. Examples include GPT (Generative Pre-trained Transformer) and similar architectures. LLMs can hold conversations, answer questions, and assist in content creation by leveraging their vast knowledge base.
The Road Ahead: A Future Shaped by Collaboration
Looking forward, the future of AI is intertwined with both great promise and significant challenges. We can expect smarter, more autonomous systems that operate seamlessly alongside humans. Innovations like general AI (machines that can perform any intellectual task a human can) remain the ultimate goal, though they are still beyond our current grasp.
However, with great power comes the responsibility to use it ethically. The AI of tomorrow will need robust regulations and guidelines to ensure fairness, transparency, and the protection of human rights. AI governance will play a crucial role in establishing frameworks that guide the development and deployment of AI technologies responsibly. This involves creating policies that manage risks, promote equitable outcomes, and prevent misuse while fostering innovation. Collaboration between researchers, policymakers, and industry leaders will be crucial to navigate this new era.
Conclusion: A Story Still Unfolding
The story of AI is one of vision, setbacks, breakthroughs, and continuous evolution. From the speculative thoughts of ancient philosophers to today’s cutting-edge technologies, AI has come a long way—and it still has a long road ahead. The future of AI holds immense potential, not just for technological growth, but for reshaping society in ways we are only beginning to imagine.
As we stand on the brink of this next chapter, the question is not just what AI will achieve, but how we will harness its power to build a future that uplifts and benefits the society as a whole.